Comparison of machine learning parametric and non-parametric techniques for determining soil moisture: Case study at las palmas andean basin
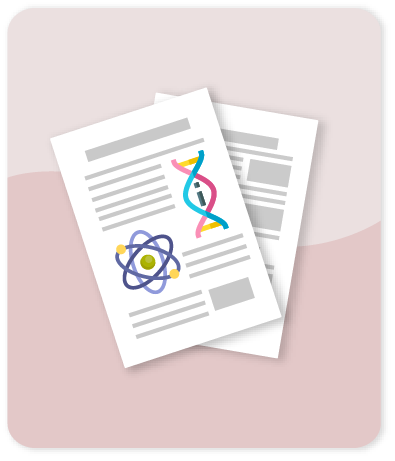
Compartir este ítem
Fecha
2021Autor
López-Bermeo C
González-Palacio M
Sepúlveda-Cano L
Montoya-Ramírez R
Hidalgo-Montoya C.
Citación
Metadatos
Mostrar el registro completo del ítemResumen
Soil moisture is one of the most important variables to monitor in agriculture. Its analysis gives insights about strategies to utilize better a particular area regarding its use, i.e., pasture for cows (or similar), production forests, or even to answer what crops should be planted. The vertical structure of the soil moisture plays an important role in several physical processes such as vegetation growth, infiltration process, soil – atmosphere interactions, among others. Despite a set of tools are currently being evaluated and used to monitor soil moisture, including satellite images and in-situ sensor, several drawbacks are still persisting. In situ data is expensive for high spatial monitoring and vertical measurements and satellite data have low spatial resolution and only retrieval information of soil moisture for the top few centimeters of the soil. The present work shows an experiment design for collecting soil moisture data in a specific Andean basin with in-situ sensors in different kinds of soils as a promising tool for reproducing soil moisture profiles in areas with scarce information, employing only surface soil moisture and simple soil characteristics. Collected data is used to train machine learning supervised parametric (Multiple Linear Regression - MLR) and non-parametric models (Artificial Neural Networks - ANNs and Support Vector Regression - SVR) for soil moisture estimation in different depths. Conclusions show that parametric methods do not meet goodness of fit assumptions; so, non-parametric methods must be considered, and SVR outperforms parametric methods regarding regression accuracy allowing to reproduce the soil moisture content profiles. The proposed SVR model represents a high potential tool to replicate the soil moisture profiles using only surface information from remote sensing or in-situ data. © 2021 ASTES Publishers. All rights reserved.
Colecciones
- Indexados Scopus [1813]